What is artificial intelligence?
2024-06-10 / tech
Malicious words, insults, and other disagreements on the internet that may seem trivial but can change the world.
Artificial Intelligence (AI) is sexy, AI is cool. AI is exacerbating inequality, upending job markets, and disrupting education. AI is a theme park ride, AI is magic. AI is our last invention, AI is a moral imperative. AI is the buzzword of the decade, AI is a marketing term from 1955. AI is like humans, AI is alien. AI is super smart, yet as dumb as dirt. The AI craze will boost the economy, the AI bubble is about to burst. AI will increase prosperity and enable humans to thrive to the fullest extent in the universe. AI will kill us all.
What on earth are people talking about?
AI is the hottest technology of the moment. But what exactly is it? This question may sound silly, yet it is the most pressing issue that people need to address. The short answer is: AI is an umbrella term referring to a set of technologies that enable computers to perform tasks that people believe require human intelligence. Such as recognizing faces, understanding speech, driving cars, writing sentences, answering questions, creating images. But even this definition encompasses a lot.
Advertisement
Herein lies the problem. What does it mean for machines to understand speech or write sentences? What tasks can we assign to these machines? How much should we trust machines to perform these tasks?
As this technology moves from prototype to product at an ever-increasing pace, these questions have become everyone's questions. But (spoiler alert!) I don't have the answers. I can't even tell you what AI is. The people who make AI don't know what AI is. Actually, they don't. "These questions are very important, and everyone feels they can have an opinion," says Chris Olah, Chief Scientist at San Francisco AI lab Anthropic. "I also think you can argue about this issue as you please, and there is no evidence to contradict your viewpoint right now."
But if you are willing to buckle up and embark on a journey, I can tell you why no one really knows, why everyone seems to disagree, and why it's right that you care.
Let's start with a casual joke.
In 2022, halfway through the first episode of "The Hype AI Theater 3000," a podcast that's a bummer, the grumpy co-hosts Alex Hanna and Emily Bender amusingly poke "the sharpest needles" into some of Silicon Valley's most hyped sacred cows. They read with vitriol an absurd 12,500-word article by Google Engineering VP Blaise Agüera y Arcas published on Medium titled "Can Machines Learn How to Behave?" Agüera y Arcas suggests that AI can understand concepts in a way somewhat similar to how humans understand concepts—such as moral values. In short, perhaps machines can be taught how to behave.Hannah and Bender dismiss this with a wave of their hands. They decide to replace the term "artificial intelligence" with the word "mathematics"— you know, a whole lot of math.
This irreverent remark aims to dismantle the pomp and personification in the sentence they have in their sights. Soon, sociologist and director of research at the Distributed Artificial Intelligence Research Institute, Hannah, and computational linguist at the University of Washington, Bender (also a well-known critic of tech industry hype on the internet), expose the chasm between what Aquila Acas wants to convey and the way they choose to listen.
"How should artificial intelligence, its creators, and users take on moral responsibility?" asks Agüera y Arcas.
Bender inquires, "How should mathematics take on moral responsibility?"
"There is a categorical mistake here," she says. Hannah and Bender not only reject Aquila Alcas's claim but also assert that it is nonsensical. "Can we stop using 'an artificial intelligence' or 'artificial intelligence' as if they are individuals in the world?" says Bender.
It sounds as if they are talking about different things, but that is not the case. Both sides are discussing large language models, the technology behind the current artificial intelligence craze. It's just that the way we talk about artificial intelligence is more polarized than ever before. In May of this year, OpenAI CEO Sam Altman revealed on Twitter the latest update of the company's flagship model GPT-4, "It feels like magic to me."
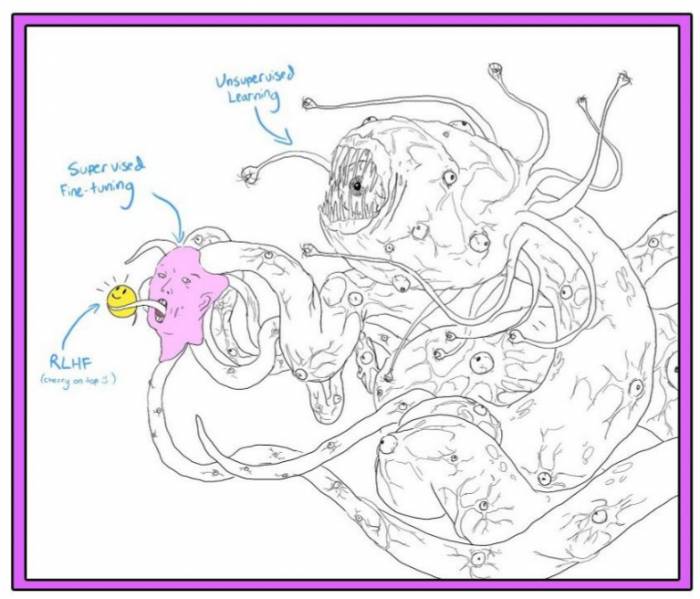
There is still a long way to go between mathematics and magic.
Artificial intelligence has followers who firmly believe in the current power of the technology and the inevitable improvements of the future. They say, general artificial intelligence is coming; super intelligence is coming. There are also heretics who dismiss these claims as mystical nonsense, scoffing at them.
Popular narratives are shaped by a multitude of big names, from chief marketing officers of large tech companies like Sundar Pichai and Satya Nadella, to industry leaders like Elon Musk and Altman, and to renowned computer scientists like Geoffrey Hinton. Sometimes, these supporters and detractors are the same person, telling us the technology is too good, but actually, it's bad.
As artificial intelligence hype continues to rise, a group opposed to the hype begins to stand up against it, ready to challenge its ambitious and often absurd claims. Supporting this direction are a large number of researchers, including Hannah and Bender, as well as outspoken industry critics, such as influential computer scientist and former Google employee Timnit Gebru and New York University cognitive scientist Gary Marcus. They all have a large following responding to them.In short, artificial intelligence has become an indispensable part in everyone's eyes, dividing the field into different camps. It feels as if different camps are talking past each other, not always with the best of intentions.
Perhaps you find all of this silly or tiresome. But considering the power and complexity of these technologies—they have been used to determine how much we pay for insurance, how we find information, how we work, and so on—it is time for us to at least agree on what we are talking about.
However, in all my conversations with those at the cutting edge of this technology, no one directly answers what they are actually building. (A brief note: This article focuses on the AI debates in the United States and Europe, mainly because that is where many of the most well-funded, cutting-edge AI labs are. But of course, there is important research elsewhere, and these countries have varying perspectives on artificial intelligence, especially China.) Part of the reason is the pace of development. But science is also open. Today's large language models can create astonishing things. The field simply cannot find common ground on what is really happening behind the scenes.
These models are trained to complete sentences. They seem capable of doing more—solving high school math problems, writing computer code, passing law exams, composing poetry. When a human does these things, we consider it a sign of intelligence. So what about when a computer does these things? Does it seem intelligent enough?
These questions touch on the core of what we call "artificial intelligence," a term that has been debated for decades. But as large language models rise, the discussion around AI becomes more intense, with these models mimicking our speech and writing in an exciting/chilling (depending on the situation) sense of realism.
We have created machines with human-like behaviors, but we have not yet shaken the habit of imagining human-like thinking behind them. This leads to an overestimation of AI's capabilities; it solidifies intuitive reactions into dogmatic positions and exacerbates the broader cultural war between technological optimists and technological skeptics.
In addition to this uncertainty, there is a significant cultural baggage, from science fiction (I bet many people in this industry grew up reading these sci-fi novels) to the more nefarious ideologies that influence our views of the future. Given this dizzying mix, the debate about artificial intelligence is no longer just academic (perhaps it never was). AI stirs passions and makes adults hurl insults at each other.
Marcus says of the debate: "The intellectual situation is not healthy." For years, Marcus has been pointing out the flaws and limitations of deep learning, the technology that has propelled AI into the mainstream, powering everything from Master of Laws to image recognition to self-driving cars. In his 2001 book "Algebraic Thinking," he pointed out that neural networks, the foundation of deep learning, cannot reason on their own. (We'll skip this topic for now, but I'll come back to it later, and we'll see how important the word "reasoning" is in such sentences.)
Marcus said he tried to engage Hinton in a formal debate about just how good large language models are, Hinton publicly expressed concerns last year about the technology he helped invent. Marcus said: "He just wouldn't do it. He called me a fool." (I have spoken with Hinton about Marcus in the past and can confirm this. "ChatGPT obviously knows more about neural networks than he does," Hinton told me last year.) Marcus also caused an uproar by writing an article titled "Deep Learning is Hitting a Wall." Altman responded on Twitter: "Give me the confidence of a mediocre deep learning skeptic."
Meanwhile, Marcus's drumming has also made him a personal brand and earned him an invitation last year to sit with Altman and testify before the U.S. Senate AI Oversight Committee.This is why all these disputes are more important than the usual internet malice. Of course, it involves huge egos and vast sums of money. But more importantly, these controversies become significant when heads of state and lawmakers convene industry leaders and opinionated scientists to explain what this technology is, what it can do (and how afraid we should be). They become significant when this technology is integrated into the software we use every day, from search engines to word processing applications to smartphone assistants. Artificial Intelligence is not going away. But if we don't know what we're being sold, who is the charlatan?
"It's hard to think of another technology in history that has sparked such a debate—debating whether it's everywhere or doesn't exist at all," write Stephen Cave and Kanta Dihal in their 2023 book "Imagining AI." The book compiles essays on how different cultural beliefs affect people's views on artificial intelligence. "That people can hold such views about AI is testament to its mythic quality."
Most importantly, artificial intelligence is an idea, an ideal, influenced as much by mathematics and computer science as by worldviews and science fiction metaphors. When we talk about artificial intelligence, clarifying what we are talking about will clear up many things. We won't agree on these issues, but a consensus on what artificial intelligence is would be a good starting point to begin discussing what it should be.
In any case, what exactly are people arguing about?
At the end of 2022, shortly after OpenAI released ChatGPT, a new meme began to circulate online that captured the strangeness of the technology better than anything else. In most versions, a Lovecraftian monster named Shoggoth, all tentacles and eyes, holds up a bland smiley face emoji, as if to mask its true nature. ChatGPT performs conversational text games as approachable as a human, but behind this facade lies unfathomable complexity and horror. ("This is a horrible, indescribable thing, larger than any subway train—a formless, protoplasmic bubble of aggregation," HP Lovecraft described Shoggoth in his 1936 novella "At the Mountains of Madness.")
Dihal says that for years, one of the most famous touchstones of artificial intelligence in popular culture has been "The Terminator." But by putting ChatGPT online for free, OpenAI has allowed millions of people to experience something different firsthand. "Artificial intelligence has always been a very vague concept that can expand infinitely to encompass a variety of ideas," she says. But ChatGPT has made these ideas tangible: "Suddenly, everyone has a concrete thing to refer to." What is artificial intelligence? For millions of people, the answer is now: ChatGPT.
The AI industry is heavily promoting this smiley face. Look at the recent hype of industry leaders on "The Daily Show." Silicon Valley chief venture capitalist Marc Andreessen said, "This has the potential to make life better... I think it's really a fantastic idea." Altman said, "I don't want to sound like a utopian tech bro here, but the quality of life improvements that artificial intelligence can bring are extraordinary." Pichai said, "Artificial intelligence is the most profound technology that humanity is working on. More profound than fire."
But as the meme points out, ChatGPT is just a friendly mask. Behind it is a monster called GPT-4, a large language model built by a massive neural network that has absorbed more words than most of us could read in a thousand lifetimes. During training, these models need to fill in the blanks in sentences obtained from millions of books and a large part of the internet, and the training can last for months, costing tens of millions of dollars. They perform this task over and over again. In a sense, they are trained to be super auto-completion machines. Ultimately, the model translates a vast amount of written information from the world into statistical representations, that is, which words are most likely to follow other words, and these statistical representations encompass billions of numerical values.
This is mathematics—lots of mathematics. No one disputes this. But is it just mathematics? Or does this complex mathematics encode algorithms capable of reasoning or concept formation similar to humans?Many people who answer "yes" to this question believe that we are on the verge of unlocking something called "Artificial General Intelligence," or AGI, a hypothetical future technology that can perform various tasks as well as humans. Some of them even look to what they call superintelligence, a science fiction technology that can do better than humans. This group believes that AGI will completely change the world—but what will happen in the end? This is another point of tension. It could solve all the problems in the world—or it could bring about destruction.
Today, AGI appears in the mission statements of the world's top artificial intelligence laboratories. But the term was coined in 2007 as a niche attempt to inject some vitality into a field then known for reading handwritten content on bank deposit slips or recommending the next book. The idea was to recapture the original vision of artificial intelligence, which was to do things similar to humans (more details will be introduced later).
Shane Legg, the co-founder of Google DeepMind who created the term, told me last year that it is actually a wish: "I don't have a particularly clear definition."
AGI has become the most controversial concept in the field of artificial intelligence. Some see it as the next big thing: AGI is artificial intelligence, but you know, it's much better. Others claim that the term is too vague and meaningless.
"AGI used to be a dirty word," Ilya Sutskever told me before stepping down as Chief Scientist at OpenAI.
But large language models, especially ChatGPT, have changed everything. AGI has gone from being a dirty word to a marketing dream.
I believe this highlights one of the most illustrative controversies of the moment—it establishes the two sides of the argument and their stakes.
Witnessing the magic of machines
A few months before OpenAI's large language model GPT-4 was publicly released in March 2023, the company shared a pre-release version with Microsoft, which hoped to use the new model to revamp its search engine Bing.
At the time, Sebastian Bubeck was researching the limitations of the Master of Laws degree and had some doubts about its capabilities. Particularly, Bubeck, who was then the Vice President of Generative Artificial Intelligence Research at Microsoft Research in Redmond, Washington, had been trying to use the technology to solve middle school math problems without success. For example: x - y = 0; what are x and y? "I think reasoning is a bottleneck, a barrier," he said. "I think you have to do something completely different to overcome this barrier."Then he began to study GPT-4. The first thing he did was to try to solve those mathematical problems. "This model succeeded," he said. "It's 2024 now, of course GPT-4 can solve linear equations. But at the time, that was crazy. GPT-3 couldn't do that."
But Bubeck's true Damascus moment came when he pushed himself to do something new. The characteristic of high school math problems is that they are all over the internet, and GPT-4 might just have memorized them. "How do you study a model that may have seen everything written by humans?" Bubeck asked. His answer was to test GPT-4 with a series of problems that he and his colleagues believed to be novel.
Working with mathematician Ronen Eldan from Microsoft Research, Bubeck asked GPT-4 to provide a mathematical proof that there are infinitely many prime numbers in the form of poetry.
Here is a snippet of GPT-4's response: "If we take the smallest number in S that is not in P / and call it p, we can add it to our set, do you understand? / But this process can be repeated indefinitely. / Therefore, our set P must also be infinite, you would agree."
Cute, right? But Bubeck and Eldan thought it was much more than that. "We were right here in this office," Bubeck said, waving his hand towards the room behind him over Zoom. "Both of us fell off our chairs. We couldn't believe what we were seeing. It was so creative, and, you know, different."
The Microsoft team also used GPT-4 to generate code to add horns to a unicorn cartoon image drawn with the word processing program Latex. Bubeck believes this shows that the model can read existing Latex code, understand what it depicts, and determine where the horns should go.
"There are many examples, but a few of them are solid evidence of reasoning," he said—reasoning being an essential part of human intelligence.
Bubeck, Eldan, and other Microsoft researchers described their findings in a paper titled "Sparks of General Artificial Intelligence": "We believe that the intelligence of GPT-4 marks a true paradigm shift in the field of computer science and beyond." When Bubeck shared the paper online, he tweeted: "It's time to face reality, the sparks of #AGI have been ignited."
The Sparks paper quickly became notorious and a touchstone for AI proponents. Agüera y Arcas and Peter Norvig, former director of research at Google and co-author of the book "Artificial Intelligence: A Modern Approach" (which may be the world's most popular AI textbook), co-wrote an article titled "Artificial General Intelligence Has Arrived." The article, published in the magazine Noema, supported by the Berggruen Institute in Los Angeles, starts with the Sparks paper and posits: "Artificial General Intelligence (AGI) means many different things to different people, but its most important part has already been realized by the current generation of advanced AI large language models," they wrote. "Decades from now, they will be recognized as the first true examples of AGI."Since then, the hype has continued to escalate. Leopold Aschenbrenner, then a researcher at OpenAI and focused on superintelligence, told me last year: "The progress in artificial intelligence over the past few years has been incredibly rapid. We've been breaking all benchmarks, and this progress continues. But it won't stop there. We will have superhuman models, models much smarter than us." (He was dismissed by OpenAI in April for claiming that he raised safety issues about the technology he was developing and "annoyed some people." Since then, he has established a Silicon Valley investment fund.)
In June, Aschenbrenner released a 165-page manifesto claiming that by "2025/2026," the pace of artificial intelligence development would outstrip that of university graduates, and by 2020, "we will have true superintelligence." However, others in the industry scoffed at such claims. When Aschenbrenner posted a chart on Twitter to illustrate the pace at which he believed artificial intelligence would continue to develop given its rapid progress in recent years, tech investor Christian Keil replied, saying that by the same logic, his son doubled in weight after birth and would weigh 7.5 trillion tons by the age of 10.
The "spark of AGI" has also become a byword for exaggerated publicity, which is not surprising. "I think they're a bit carried away," Marcus said of the Microsoft team. "They're excited as if 'Hey, we've discovered something! This is amazing!' They haven't subjected it to scrutiny by the scientific community." Bender referred to the Sparks paper as "fan fiction."
Claiming that GPT-4's behavior shows signs of AGI is not only provocative, but Microsoft, which uses GPT-4 in its own products, clearly intends to promote the capabilities of the technology. A tech chief operating officer posted on LinkedIn: "This document is a marketing gimmick disguised as research."
Some also believe there are flaws in the methodology of the paper. Its evidence is hard to verify because it comes from interactions with a version of GPT-4 that is not accessible outside of OpenAI and Microsoft. Bubeck acknowledged that the public version has safeguards that limit the model's capabilities. This prevents other researchers from replicating his experiments.
A research group attempted to replicate the unicorn example using a coding language called Processing, which GPT-4 can also use to generate images. They found that the public version of GPT-4 could generate a passable unicorn but could not flip or rotate the image by 90 degrees. This may seem like a minor difference, but when you claim the ability to draw a unicorn as a sign of AGI, these things really matter.
The key to the examples in the Sparks paper (including the unicorn) is that Bubeck and his colleagues believe they are genuine examples of creative reasoning. This means the team must determine that the examples of these tasks or tasks very similar to them are not included in the vast dataset that OpenAI has accumulated to train its models. Otherwise, the results might be interpreted as instances of GPT-4 recreating patterns it has already seen.
Bubeck insists that the tasks they set for the model are not found on the internet. Drawing a cartoon unicorn with Latex is definitely one of the tasks. But the internet is a big place. Other researchers quickly pointed out that there are indeed online forums dedicated to drawing animals with Latex. "For the record, we are aware of this," Bubeck replied on X. "Every query in the Sparks paper has been thoroughly searched on the internet."
(This did not stop the insults: "I demand that you stop being a fraud," computer scientist Ben Recht from UC Berkeley replied on Twitter, then accused Bubeck of "being caught lying.")Bubeck insisted that the work was well-intentioned, but he and his co-authors admitted in their paper that their method was not rigorous—relying merely on notebook observations rather than foolproof experiments. Despite this, he had no regrets: "The paper has been published for over a year, and I haven't seen anyone provide me with a convincing argument that unicorns are not genuine examples of reasoning."
This was not to say that he could directly answer the big question—although his response revealed what kind of answer he wanted to give. "What is artificial intelligence?" Bubeck repeated. "I want to make it clear to you. The question may be simple, but the answer could be complex."
"There are still many simple questions for which we do not know the answers. Some of these simple questions are the most profound," he said. "I equate this question with where did life originate? Where did the universe originate? Where do we come from? These are all big questions."
Seeing only the mathematics in the machine
Before becoming one of the main opponents of artificial intelligence proponents, Bender left her mark in the field of artificial intelligence as a co-author of two influential papers. (She likes to point out that both papers have been peer-reviewed—unlike the Sparks paper and many other highly regarded papers.) The first paper, co-authored with computational linguist Alexander Koller from Saarland University in Germany, was published in 2020 and titled "Towards NLU" (NLU stands for natural language understanding).
"For me, it all started with arguing with others in the computational linguistics field about whether language models understand anything," she said. (Understanding, like reasoning, is generally considered a fundamental element of human intelligence.)
Bender and Koller argued that models trained solely on text could only ever learn the form of language, not its meaning. They believed that meaning consists of two parts: words (which can be tokens or sounds) plus the reasons for uttering these words. There are many reasons people use language, such as sharing information, telling jokes, flirting, warning someone to step back, and so on. Stripping away these contexts, the texts used to train LLMs like GPT-4 can enable them to mimic language patterns so well that many sentences generated by LLMs look identical to those written by humans. But there is no meaning behind them, no spark. It's an impressive statistical trick, but utterly meaningless.
They used a thought experiment to illustrate their point. Imagine two English speakers stranded on neighboring deserted islands. There is an underwater cable that allows them to send text messages to each other. Now imagine an octopus, which does not understand English but is good at statistical pattern matching. It wraps its suckers around the cable and starts eavesdropping on the messages. The octopus is so good at guessing which words follow others that when it severs the cable and starts replying to one of the islanders' messages, the islander believes she is still chatting with her neighbor. (If you haven't noticed, the octopus in this story is a chatbot.)
The person conversing with the octopus would be fooled for a reasonable amount of time, but could it last? Can the octopus understand what is being said over the phone?Joan Ionada
Imagine for a moment that the islander now says she has built a coconut catapult and asks the octopus to do the same, telling it her thoughts. The octopus cannot do this. If it does not know what the words in the information refer to in the world, it cannot follow the islander's instructions. Perhaps it might guess the answer: "Okay, great idea!" The islander might think this means the person she is talking to has understood her message. But if so, she is creating something out of nothing. Finally, imagine the islander is attacked by a bear and sends a distress signal downstream. How would the octopus handle these words?
Bender and Koller believe that this is how large language models learn and why they have limitations. "This thought experiment shows why this path will not lead us to a machine that can understand anything," says Bender. "The octopus's situation is that we gave it training data, which is a conversation between two people, and that's it. But then, suddenly, something unexpected comes up that it cannot handle because it has not understood."
Another famous paper by Bender is "The Dangers of Stochastic Parrots," which emphasizes a series of hazards that she and her co-authors believe companies creating large language models have overlooked. These include the huge computational cost of creating models and their environmental impact; the deeply ingrained racism, sexism, and other abusive language in the models; and the dangers of creating a system that can deceive humans, a system that "randomly concatenates sequences of linguistic forms... based on the probability information of how they combine, without reference to any meaning: a stochastic parrot."
Google's higher-ups were not pleased with this paper, and the resulting conflict led to Bender's two co-authors, Timnit Gebru and Margaret Mitchell, being forced to leave the company, where they had led the AI ethics team. This also made "stochastic parrot" a popular pejorative term for large language models—Bender also found herself in a whirlpool of abuse.
For Bender and many like-minded researchers, the bottom line is that the field has been bewitched: "I think they are being led to imagine entities with autonomous thought, entities that can make decisions for themselves and ultimately become truly accountable for these decisions."
Bender has always been a linguist, and she told me that she won't even use the term "AI" now "without quotation marks." Ultimately, for her, it is a buzzword from tech giants that can distract people from many related hazards. "I'm involved now," she says. "I care about these issues, and the hype is getting in the way."
Extraordinary Evidence?
Aquila Acas calls people like Bender "AI deniers"—implying that they will never accept what Bender takes for granted. Bender's position is that extraordinary claims require extraordinary evidence, and we do not have such evidence.
But some people are looking for it, and unless they find a clear answer—spark or stochastic parrot or something in between—they would rather sit on their hands. We call this the wait-and-see camp.Brown University's Ellie Pavlick, who studies neural networks, told me: "The idea that human intelligence can be recreated through such mechanisms is offensive to some people."
She added: "People have a strong conviction about this issue—it almost feels like a religion. On the other hand, some people have a bit of a god complex. So for them, suggesting that they can't do it is also offensive."
Pavlick is fundamentally an agnostic. She insists that she is a scientist and will follow whatever direction science leads. She dismisses the more outlandish claims, but she believes that there must be something exciting happening. "This is where I disagree with Bender and Kohler," she told me. "I think there is actually some spark—maybe not AGI, but there is something in it that we did not expect to find."
Ellie Pavlick
Courtesy photo
The problem is that people do not agree on what these exciting things are and why they are exciting. With so much hype, it's easy to become cynical.
When you listen to researchers like Bubeck, they seem much more measured. He believes that infighting overlooks the nuances of his work. "I don't think there's any problem with holding views at the same time," he said. "There is random parroting; there is reasoning—it's a spectrum. It's very complex. We don't have all the answers."
"We need a whole new vocabulary to describe what's happening," he said. "When I talk about reasoning in large language models, one of the reasons people object is that it's different from human reasoning. But I think we can't help but call it reasoning. It is reasoning."
Anthropic's Olah is very cautious when facing the LLM program, but his company is one of the hottest artificial intelligence labs in the world today, having created the Claude 3 program, which has received as much (if not more) hyperbolic praise since its release earlier this year as GPT-4.
"I feel that a lot of the discussion about the capabilities of these models is very tribal," he said. "People have preconceived notions, and there's no evidence to support either side. Then it becomes an argument based on feelings, and I think arguments based on feelings on the internet often go in the wrong direction."Euler told me that he has his own conjectures. "My subjective impression is that these things are tracking very complex ideas," he said. "We don't yet have a comprehensive story about how large models operate, but I think it's hard to reconcile what we're seeing with the extreme 'random parrot' scenario."
That's all he would say: "I don't want to speculate too much beyond what our existing evidence can strongly infer."
Last month, Anthropic released a study in which researchers equipped Claude 3 with a neural network equivalent to an MRI. By monitoring which parts of the model were activated and deactivated as it ran, they identified specific patterns of neurons that were activated when the model was presented with certain inputs.
Anthropic also reported patterns associated with inputs attempting to describe or demonstrate abstract concepts. "We saw features related to deception and honesty, flattery, security vulnerabilities, and bias," Olah said. "We found features related to the pursuit of power, manipulation, and betrayal."
These results give us the clearest insight yet into the inner workings of large language models. It allows us to see seemingly elusive human-like characteristics. But what does it really tell us? As Olah acknowledged, they don't know how the model processes these patterns. "It's a relatively limited picture, and it's quite difficult to analyze," he said.
Even though Olah won't elaborate on what he thinks is happening inside large language models like Claude 3, it's clear that the question is important to him. Anthropic is known for its work in artificial intelligence safety—ensuring that powerful future models operate in the way we want them to, rather than in ways we don't want them to (known in industry jargon as "alignment"). Figuring out how today's models work is not only a necessary first step in controlling future models; it also tells you how worried you need to be about doomsday scenarios first. "If you think the models won't be very powerful," Olah said, "then they probably won't be very dangerous."
Why We Can't Get Along
In 2014, in a BBC interview looking back on her career, influential cognitive scientist Margaret Boden, now 87, was asked if she thought there were certain limitations preventing computers (which she referred to as "tin cans") from doing what humans can do.
"I certainly don't think there's anything in principle," she said. "Because to deny that would be to say that [human thought] happens by magic, and I don't believe it happens by magic."
But she warned that powerful computers are not enough to achieve this goal: the field of artificial intelligence also needs "powerful ideas"—new theories about how thinking happens and new algorithms that might replicate thinking. "But these things are very, very difficult, and I don't think there's any reason to assume that we will one day be able to answer all these questions. Maybe we will, maybe we won't."Boden is reflecting on the early days of the current boom, but this "will we, won't we" vacillation indicates that for decades, she and her peers have been grappling with the same conundrums that researchers face today. Artificial Intelligence (AI) began with an ambitious aspiration over 70 years ago, and we still disagree on what can be achieved, what cannot, and how to know if we have achieved the goal. Most, if not all, of these disputes boil down to: we have yet to fully understand what intelligence is, or how to recognize it. The field is rife with speculation, yet no one can say for certain.
Ever since the concept of AI began to be taken seriously, we have been stuck on this issue. Even before that, when the stories we consumed began to deeply embed the concept of humanoid machines into our collective imagination. The long history of these disputes means that today's struggles often exacerbate the divisions that have existed from the outset, making it harder for people to find common ground.
To understand how we got to this point, we need to understand the path we have taken. So, let's delve into the origin story of AI—which was also heavily hyped for profit.
A Brief History of the AI Spin
Computer scientist John McCarthy coined the term "Artificial Intelligence" in 1955 while writing a funding proposal for a summer research project at Dartmouth College in New Hampshire.
The plan was for McCarthy and a small group of fellow researcher colleagues (a who's who of post-war American mathematicians and computer scientists)—or, in the words of Harry Law, a researcher at the University of Cambridge studying the history of AI and ethics and policy at Google DeepMind, "John McCarthy and his gang"—to come together for two months (not a typo) to make significant progress on the new research challenge they had set for themselves.
From left to right, Oliver Selfridge, Nathaniel Rochester, Ray Solomonoff, Marvin Minsky, Peter Milner, John McCarthy, and Claude Shannon sit on the lawn at the 1956 Dartmouth Conference.
McCarthy and his co-authors wrote: "The study is based on the supposition that every aspect of learning or any other feature of intelligence can be so precisely described that a machine can simulate it." "We will attempt to find how to make machines use language, form abstractions and concepts, solve various problems now only solvable by humans, and improve themselves."
Their wish list of what they hoped machines could do—Bender calls it the "unrealistic dream"—has not changed much. Using language, forming concepts, and solving problems are the defining goals of today's AI. Their hubris has not changed much either: "We think that significant progress can be made in one or more of these problems if a carefully selected group of scientists spend a summer working on them," they wrote. Of course, that summer has now lasted seventy years. And the extent to which these problems have actually been solved is still a matter of loud debate on the internet.
However, what is often overlooked in this classic history is that AI was hardly ever called "Artificial Intelligence."More than one of McCarthy's colleagues disliked the term he proposed. Historian Pamela McCorduck, in her 2004 book "Machines Who Think," quoted Arthur Samuel, a participant at Dartmouth and the creator of the first computer to play checkers: "The term 'artificial intelligence' gives the impression that there's something bogus about it." Claude Shannon, a co-author of the Dartmouth proposal and sometimes referred to as the "father of the information age," preferred the term "automata studies." Two other AI pioneers, Herbert Simon and Allen Newell, continued to refer to their work as "complex information processing" for many years thereafter.
In fact, "artificial intelligence" was just one of several labels that might encompass the diverse ideas the Dartmouth team drew upon. Historian Jonnie Penn has identified alternative terms that might have been in use at the time, including "engineering psychology," "applied epistemology," "neuro-cybernetics," "non-numerical computation," "neurodynamics," "advanced automatic programming," and "hypothetical automata." This list reveals how varied the inspirations for their new field were, encompassing biology, neuroscience, statistics, and more. Another Dartmouth participant, Marvin Minsky, described AI as a "suitcase word" because it could accommodate so many different interpretations.
But McCarthy wanted a name that would reflect his ambitious vision. Calling this new field "artificial intelligence" drew attention and money. Don't forget: artificial intelligence was sexy, it was cool.
Beyond the terminology, the Dartmouth proposal also established the divisions between opposing approaches in the field of artificial intelligence, which have plagued the field ever since—Lau calls this division "the core contradiction of artificial intelligence."
McCarthy and his colleagues hoped to describe "every aspect of learning or any other intelligent characteristic" in computer code so that machines could mimic them. In other words, if they could figure out how the mind worked—reasoning rules—and write down the recipe, they could program computers to follow it. This laid the groundwork for what later became known as rule-based or symbolic artificial intelligence (sometimes now referred to as GOFAI, "Good Old-Fashioned AI"). But the process of proposing hard-coded rules to capture the problem-solving process for real, non-trivial problems proved to be too difficult.
Another path leaned towards neural networks, computer programs that would try to learn these rules on their own in the form of statistical patterns. The Dartmouth proposal barely mentioned this in passing (referring to "neuron nets" and "neural nets"). Although the idea initially seemed less promising, some researchers continued to explore versions of neural networks and symbolic AI. But it would take several more decades—plus a lot of computing power and a wealth of data on the internet—for them to truly take off. Fast forward to today, and this approach underpins the entire boom in artificial intelligence.
The big takeaway here is that, much like today's researchers, the innovators of artificial intelligence also argued over foundational concepts and got caught up in their own hype. Even the GOFAI team was not immune to this. Aaron Sloman, a philosopher and a pioneer in AI, now in his 80s, recalls knowing Minsky and McCarthy in the 70s when they "disagreed": "Minsky thought McCarthy's logic wouldn't work, and McCarthy thought Minsky's mechanisms couldn't do what logic could. I got on well with both of them, but I said at the time, 'Neither of you has got it right.'" (Sloman still believes no one has explained how human reasoning uses intuition like logic, but that's another digression!)
As the technology waxed and waned, the term "AI" also went in and out of fashion. In the early 1970s, the British government released a report stating that the AI dream was hopeless and not worth funding, effectively shelving these two research paths. In fact, all the hype was to no avail. Research projects were shut down, and computer scientists removed the term "artificial intelligence" from their funding proposals.
In 2008, when I completed my Ph.D. in computer science, there was only one person in the department researching neural networks. Bender has a similar recollection: "When I was in college, a popular joke was that artificial intelligence was everything we hadn't figured out how to do with computers yet. Like, once you figure out how to do it, it's no longer magic, so it's not AI."
But that magic—the grand vision proposed in the Dartmouth proposal—still exists, and as we now see, it laid the groundwork for the dream of AGI.Good Behavior and Bad Behavior
In 1950, five years before McCarthy began discussing artificial intelligence, Alan Turing published a paper posing a question: Can machines think? To answer this question, the renowned mathematician proposed a hypothetical test, which he called the imitation game. The setup imagined a person and a computer behind a screen, with another person inputting questions to each. Turing claimed that if the questioner could not distinguish which answers came from a human and which from a computer, then it could be said that the computer is thinking.
Unlike McCarthy's team, Turing believed that thinking is a difficult thing to describe. The Turing Test was a way to sidestep this issue. "He essentially said: I will not focus on the nature of intelligence itself, but rather look for its manifestations in the world. I am looking for its shadow," said Law.
In 1952, the British Broadcasting Corporation convened a panel to further explore Turing's ideas. Joining Turing in the studio were two of his colleagues from the University of Manchester—Maxwell Newell, a professor of mathematics, and Geoffrey Jefferson, a professor of neurosurgery—as well as Richard Braithwaite, a philosopher of science, ethics, and religion from the University of Cambridge.
Braithwaite began by saying, "thinking is generally considered a human specialty, perhaps also a specialty of other higher animals, this question seems too absurd to be worth discussing. But of course, it all depends on what 'thinking' includes."
The panel members discussed Turing's question but never reached a final conclusion.
As they tried to define what thinking involves and what the mechanism of thinking is, the goal shifted. "Once people can see the operation of causal relationships in the brain, they will think this is not thinking, but a lack of imagination," said Turing.
The problem was that when one panel member proposed a behavior that might be seen as evidence of thought (such as reacting angrily to a new idea), another panel member would point out that a computer could also do this.
As Newell said, it is easy to program a computer to print "I do not like this new program." But he admitted that this is just a trick.
Indeed, Jefferson said: he wanted a computer that could print "I do not like this new program" because it did not like the new program. In other words, for Jefferson, behavior was not enough. What mattered was the process that led to the behavior.But Turing disagreed. As he pointed out, uncovering a specific process—what he called the "donkey work"—does not clarify what thinking is. So what is left?
Turing said: "From this point of view, one might be inclined to define thinking as consisting of those psychological processes which we do not understand. If this is correct, then making a thinking machine is making a machine which does interesting things without our really understanding how it does them."
It feels strange to hear people argue endlessly about these ideas for the first time. "This debate is quite prescient," says Tomer Ullman, a cognitive scientist at Harvard University. "Some of the points are still alive, perhaps even more alive. They seem to be reiterating that the Turing Test is, first and foremost, a behaviorist test."
For Turing, intelligence was hard to define but easy to recognize. He believed that the appearance of intelligence was enough—but did not specify how this behavior should be generated.
However, most people, when under pressure, intuitively judge what is intelligent and what is not. There are foolish ways and clever ways to exhibit intelligence. In 1981, New York University philosopher Ned Block pointed out that Turing's proposal defies intuition. Since it does not explain the cause of this behavior, the Turing Test can be circumvented by trickery (as Newman pointed out in a BBC broadcast).
"Does a machine really think or possess intelligence, depending on the gullibility of the human questioner?" Block asked. (Or as computer scientist Mark Riedl put it: "The Turing Test is not designed for AI to pass, but for humans to fail.")
Block said, imagine a huge lookup table where human programmers have input all the answers to possible questions. Input a question to this machine, and it will search the database for a matching answer and send it back. Block believed that anyone using this machine would consider its behavior intelligent: "But in reality, the intelligence of this machine is like that of a toaster," he wrote. "All the intelligence it displays comes from its programmers."
Block concluded that whether behavior is intelligent depends on how it is generated, not how it appears. Block's toaster, later referred to as the "blockhead," is one of the most powerful counterexamples to the assumptions behind Turing's proposal.
Delve deeper
The Turing Test was not intended to be a practical metric, but its influence is deeply ingrained in our views on artificial intelligence today. This has become particularly important with the surge of master's degree programs in law over the past few years. These models are ranked based on their outward behavior, specifically their performance in a series of tests. When OpenAI announced GPT-4, it released an impressive scorecard detailing the model's performance in a variety of high school and professional exams. Almost no one talks about how these models achieve these results.That is because we do not know. Today's large language models are so complex that no one can exactly say how their behaviors are generated. Researchers outside of the few companies that make these models do not know what is in their training data; no model maker has shared the details. This makes it difficult for us to clarify what is memory and what is not memory—a random parroting. But even internal researchers like Olah do not know exactly what happens when faced with a robot obsessed with bridge.
This leaves an unresolved question: Yes, large language models are built on mathematics—but can they do something intelligent with mathematics?
The debate begins again.
Pavlik from Brown University says, "Most people try to solve problems with empty talk." This means they are just arguing about theories without considering what is really happening. "Some people would say, 'I think it is so,' while others would say, 'Well, I don't think so.' We are stuck in a deadlock."
Comment